Not all ‘open source’ AI models are actually open: here’s a ranking

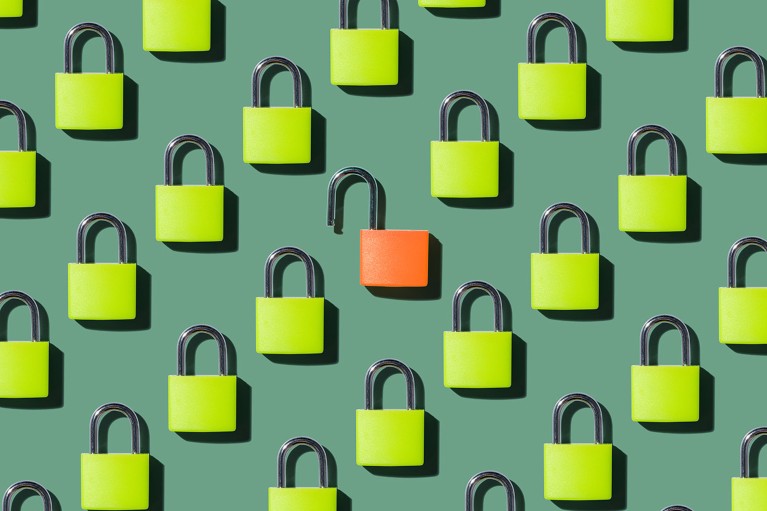
Truly open-source models should allow researchers to replicate and interrogate them.Credit: MirageC/Getty
Technology giants such as Meta and Microsoft are describing their artificial intelligence (AI) models as ‘open source’ while failing to disclose important information about the underlying technology, say researchers who analysed a host of popular chatbot models.
The definition of open source when it comes to AI models is not yet agreed, but advocates say that ’full’ openness boosts science, and is crucial for efforts to make AI accountable. What counts as open source is likely to take on increased importance when the European Union’s Artificial Intelligence Act comes into force. The legislation will apply less strict regulations to models that are classed as open.
Some big firms are reaping the benefits of claiming to have open-source models, while trying “to get away with disclosing as little as possible”, says Mark Dingemanse, a language scientist at Radboud University in Nijmegen, the Netherlands. This practice is known as open-washing.
“To our surprise, it was the small players, with relatively few resources, that go the extra mile,” says Dingemanse, who together with his colleague Andreas Liesenfeld, a computational linguist, created a league table that identifies the most and least open models (see table). They published their findings on 5 June in the conference proceedings of the 2024 ACM Conference on Fairness, Accountability and Transparency1.
The study cuts through “a lot of the hype and fluff around the current open-sourcing debate”, says Abeba Birhane, a cognitive scientist at Trinity College Dublin and adviser on AI accountability to Mozilla Foundation, a non-profit organization based in Mountain View, California.
Defining openness
The term ’open source’ comes from software, where it means access to source code and no limits on a program’s use or distribution. But given the complexity of large AI models and the huge volumes of data involved, making them open source is far from straightforward, and experts are still working to define open-source AI. Revealing all facets of a model is not always desirable for companies, because it can expose them to commercial or legal risks, says Dingemanse. Others argue that releasing models completely freely risks misuse.
What the EU’s tough AI law means for research and ChatGPT
But being labelled as open source can also bring big benefits. Developers can already reap public-relations rewards from presenting themselves as rigorous and transparent. And soon there will be legal implications. The EU’s AI Act, which passed this year, will exempt open-source general-purpose models, up to a certain size, from extensive transparency requirements, and commit them to lesser and as-yet-undefined obligations. “It’s fair to say the term open source will take on unprecedented legal weight in the countries governed by the EU AI Act,” says Dingemanse.
In their study, Dingemanse and Liesenfeld assessed 40 large language models — systems that learn to generate text by making associations between words and phrases in large volumes of data. All these models claim to be ‘open source’ or ‘open’. The pair made an openness league table by assessing models on 14 parameters, including the availability of code and training data, what documentation is published and how easy the model is to access. For each parameter, they judged whether the models were open, partially open or closed.
This sliding-scale approach to analysing openness is a useful and practical one, says Amanda Brock, chief executive officer of OpenUK, a London-based not-for-profit company that focuses on open technology.
The researchers found that many models that claim to be open or open source — including Llama from Meta and Google DeepMind’s Gemma — are, in fact, just ‘open weight’. This means that outside researchers can access and use the trained models, but cannot inspect or customize them. Nor can they fully understand how they were fine-tuned for specific tasks; for example, using human feedback. “You don’t give a lot away … then you get to claim openness credits,” says Dingemanse.
Open-source AI chatbots are booming — what does this mean for researchers?
Particularly worrying, say the authors, is the lack of openness about what data the models are trained on. Around half of the models that they analysed do not provide any details about data sets beyond generic descriptors, they say.
A Google spokesperson says that the company is “precise about the language” it uses to describe models, choosing to label its Gemma LLM as open rather than open source. “Existing open-source concepts can’t always be directly applied to AI systems,” they added. Microsoft tries to be “as precise as possible about what is available and to what extent”, a spokesperson says. “We choose to make artifacts like models, code, tools, and datasets publicly available because the developer and research communities have an important role to play in the advancement of AI technology.” Meta did not respond to a request for comment from Nature.
Models made by smaller firms and research groups tended to be more open than those of their big-tech counterparts, the analysis found. The authors highlight BLOOM, built by an international, largely academic collaboration, as an example of truly open-source AI.
Peer review ‘out of fashion’
Scientific papers detailing the models are extremely rare, the pair found. Peer review seems to have “almost completely fallen out of fashion”, being replaced by blog posts with cherry-picked examples, or corporate preprints that are low on detail. Companies “might release a nice, flashy looking paper on their website, which all looks very technical. But if you pore over it, there is no specification whatsoever of what data went into that system”, says Dingemanse.
It is not yet clear how many of these models will fit the EU’s definition of open source. Under the act, this would refer to models that are released under a “free and open” licence that, for example, allows users to modify a model but says nothing about access to training data. Refining this definition will probably form “a single pressure point that will be targeted by corporate lobbies and big companies”, the paper says.
Can we open the black box of AI?
And openness matters for science, says Dingemanse, because it is essential for reproducibility. “If you can’t reproduce it, it’s a hard sell to call it science,” he says. The only way for researchers to innovate is by tinkering with models, and to do this they need enough information to build their own versions. Not only that, but models must be open to scrutiny. “If we cannot look inside to know how the sausage is made, we also don’t know whether to be impressed by it,” Dingemanse says. For example, it might not be an achievement for a model to pass a particular exam if it was trained on many examples of the test. And without data accountability, no one knows whether inappropriate or copyrighted data has been used, he adds.
Liesenfeld says that the pair hope to help fellow scientists to avoid “falling into the same traps we fell into”, when looking for models to use in teaching and research.