Google AI has better bedside manner than human doctors — and makes better diagnoses

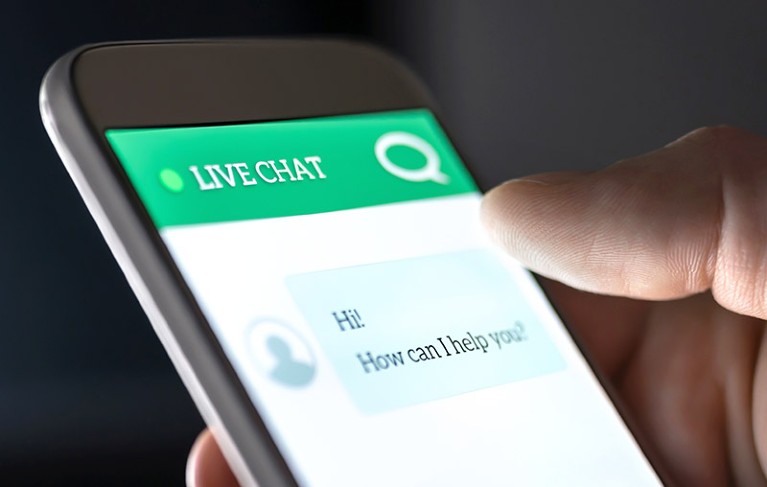
A chatbot based on Google’s large language model can take medical histories from simulated patients and offer a range of diagnoses.Credit: Tero Vesalainen/Getty
An artificial intelligence (AI) system trained to conduct medical interviews matched or even surpassed human doctors’ performance at conversing with simulated patients and listing possible diagnoses on the basis of the patients’ medical history1.
The chatbot, which is based on a large language model (LLM) developed by Google, was more accurate than board-certified primary-care physicians in diagnosing respiratory and cardiovascular conditions, among others. Compared with human doctors, it managed to acquire a similar amount of information during medical interviews and ranked higher on empathy.
Is the world ready for ChatGPT therapists?
“To our knowledge, this is the first time that a conversational AI system has ever been designed optimally for diagnostic dialogue and taking the clinical history,” says Alan Karthikesalingam, a clinical research scientist at Google Health in London and a co-author of the study1, which was published on 11 January in the arXiv preprint repository. It has not yet been peer reviewed.
Dubbed Articulate Medical Intelligence Explorer (AMIE), the chatbot is still purely experimental. It hasn’t been tested on people with real health problems — only on actors trained to portray people with medical conditions. “We want the results to be interpreted with caution and humility,” says Karthikesalingam.
Even though the chatbot is far from use in clinical care, the authors argue that it could eventually play a role in democratizing health care. The tool could be helpful, but it shouldn’t replace interactions with physicians, says Adam Rodman, an internal medicine physician at Harvard Medical School in Boston, Massachusetts. “Medicine is just so much more than collecting information — it’s all about human relationships,” he says.
Learning a delicate task
Few efforts to harness LLMs for medicine have explored whether the systems can emulate a physician’s ability to take a person’s medical history and use it to arrive at a diagnosis. Medical students spend a lot of time training to do just that, says Rodman. “It’s one of the most important and difficult skills to inculcate in physicians.”
One challenge facing the developers was a shortage of real-world medical conversations available to use as training data, says Vivek Natarajan, an AI research scientist at Google Health in Mountain View, California, and a co-author of the study. To address that challenge, the researchers devised a way for the chatbot to train on its own ‘conversations’.
Generative AI could revolutionize health care — but not if control is ceded to big tech
The researchers did an initial round of fine-tuning the base LLM with existing real-world data sets, such as electronic health records and transcribed medical conversations. To train the model further, the researchers prompted the LLM to play the part of a person with a specific condition, and that of an empathetic clinician aiming to understand the person’s history and devise potential diagnoses.
The team also asked the model to play one more part: that of a critic who evaluates the doctor’s interaction with the person being treated and provides feedback on how to improve that interaction. That critique is used to further train the LLM and generate improved dialogues.
To test the system, researchers enlisted 20 people who had been trained to impersonate patients, and got them to have online text-based consultations — both with AMIE and with 20 board-certified clinicians. They were not told whether they were chatting with a human or a bot.
The actors simulated 149 clinical scenarios and were then asked to evaluate their experience. A pool of specialists also rated the performance of AMIE and that of the physicians.
AMIE aces the test
The AI system matched or surpassed the physicians’ diagnostic accuracy in all six medical specialties considered. The bot outperformed physicians in 24 of 26 criteria for conversation quality, including politeness, explaining the condition and treatment, coming across as honest, and expressing care and commitment.
“This in no way means that a language model is better than doctors in taking clinical history,” says Karthikesalingam. He notes that the primary-care physicians in the study were probably not used to interacting with patients via a text-based chat, which might have affected their performance.
By contrast, an LLM has the unfair advantage of being able to quickly compose long and beautifully structured answers, Karthikesalingam says, allowing it to be consistently considerate without getting tired.
Wanted: unbiased chatbot
An important next step for the research, he says, is to conduct more-detailed studies to evaluate potential biases and ensure that the system is fair across different populations. The Google team is also starting to look into the ethical requirements for testing the system with humans who have real medical problems.
Daniel Ting, a clinician AI scientist at Duke-NUS Medical School in Singapore, agrees that probing the system for biases is essential to make sure that the algorithm doesn’t penalize racial groups that are not well represented in the training data sets.
Chatbot users’ privacy is also an important aspect to be considered, Ting says. “For a lot of these commercial large language model platforms right now, we are still unsure where the data is being stored and how it is being analysed,” he says.